Posted: December 2, 2022
Team develops algorithms to predict yields under various scenarios.
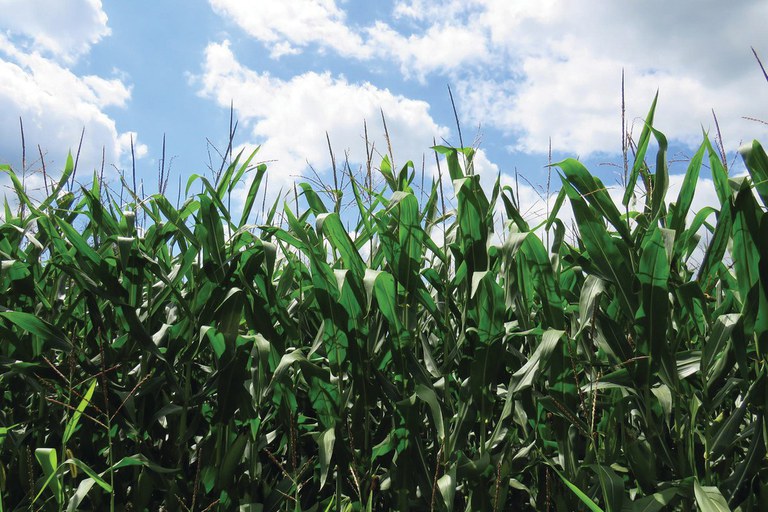
Research supported by a plant pathologist in Penn State's College of Agricultural Sciences suggests that machine-learning algorithms could increase corn and soybean yields. Credit: Pexels
Machine-learning algorithms that are programmed to recognize changing weather patterns could show producers and agricultural managers how to increase soybean and corn yields in the United States, according to new research published in Scientific Reports.
The approach could prove valuable in addressing climate change realities that have presented challenges in growing enough food for a rising global population, noted Paul Esker, associate professor of epidemiology and field crop pathology.
"Soybean and corn are among the most valuable crops in terms of food supply and economic output in the U.S. agricultural sector," said Esker, who pointed to U.S. Department of Agriculture statistics that place corn as the most widely produced crop in the United States, with soybean following close behind.
Not only are these crops vital to food security in the United States and beyond, but their combined total value to the nation's economy is more than $100 billion. While Esker acknowledges this is an impressive figure, he points out that many scientists predict that by 2050, the world must feed 9 billion people, so current outputs must increase.
Growers have access to a vast amount of crop yield and management information that has emerged from ongoing agricultural experiments conducted across the United States. Even with that knowledge, predictions are challenging because various factors interact with each other.
To overcome these challenges, the team explored the idea of leveraging the power of machine-learning algorithms. A machine-learning algorithm, Esker explained, is the method by which the computer learns from the data. The goal is to predict output values from existing input data.
To that end, the team pored over crop yield and management data from variety trials conducted in 28 states between 2016 and 2018 for corn and between 2014 and 2018 for soybeans. Information was arranged based on soil type and management practices, including irrigation, tillage method, seeding rate, row spacing, and cultivar maturity.
Additionally, weather data were retrieved for each year for each set of coordinates. The team examined correlations among the weather variables and determined seven weather variables for corn and eight for soybean for specific regions.
For each crop, the researchers developed algorithms, or data sets, which they applied to various simulations and tested over two growing seasons in a randomly chosen field in southcentral Wisconsin. Boxplots, a standardized way of displaying the distribution of data, were used to visually evaluate the results.
For that specific field and cropping system, use of the team's algorithms showed that corn with a May 1 sowing date realized a 6-percent increase in yield compared to corn planted on June 1. By creating scenarios with 256 background cropping system choices, the resultant algorithm-derived yield estimate difference for the same sowing date was smaller but still positive.
In the case of soybean, a May 1 sowing resulted in a 14-percent increase in yield compared to a June 1 sowing in the single-background cropping system. The result was consistent when yield differences due to sowing date were averaged across 128 background cropping system choices.
The results suggest that the developed algorithms have the potential to lead the agriculture industry to substantial yield increases.
--Amy Duke
Features
Fostering Forests
Across the United States, forests face unprecedented threats, and scientists in Penn State's College of Agricultural Sciences are conducting novel and complex research to conserve them.
Buzzing With Purpose
Community scientists work to protect Pennsylvania's wild bees
Conservation Reimagined
Exploring new approaches to cope with a changing climate